Viktor Artiushenko
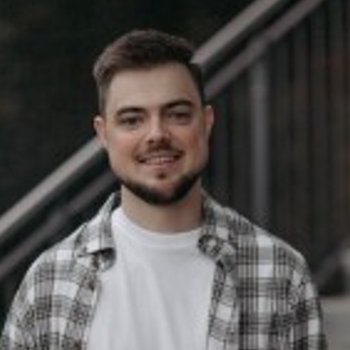
Junior Professorship for »Artificial Intelligence (AI) - Application in Production and Logistics«
Current projects
AI Engineering - An interdisciplinary, project-oriented bachelor's degree program with a focus on artificial intelligence and engineering.
Duration: 01.12.2021 bis 30.11.2025
AI Engineering (AiEng) encompasses the systematic design, development, integration and operation of solutions based on artificial intelligence (AI) using engineering methods as a model. At the same time, AiEng builds a bridge between basic research on AI methods and the engineering sciences and makes the use of AI systematically accessible and available there. The project focuses on the nationwide development of an "AI Engineering" Bachelor's degree program, which combines the training of AI methods, models and technologies with those of engineering sciences. AiEng is to be designed as a cooperative study program between Otto von Guericke University (OVGU) Magdeburg and the four universities in Saxony-Anhalt: Anhalt University of Applied Sciences, Harz University of Applied Sciences, Magdeburg-Stendal University of Applied Sciences and Merseburg University of Applied Sciences. The interdisciplinary degree program will enable students to develop AI systems and services in the industrial environment and beyond and to provide holistic support for the associated engineering process - from problem analysis to commissioning and maintenance / servicing. The AiEng curriculum provides comprehensive AI training, supplemented by basic engineering training and in-depth training in a selected application domain. In order to achieve a symbiosis of AI and engineering education, a new action-oriented framework is developed and taught, which describes the complete engineering process of AI solutions and methodically supports all phases. AIEng is characterized by a cross-module interlocking of teaching and learning content within a semester as well as by a cross-faculty and cross-university tandem teaching concept and pursues a student-centered didactic concept, which is supported by many practice-oriented (team) projects and a wide range of Open Educational Resources (OERs) with an (e)-tutor program.
Completed projects
24401_ SENECA - Development of a self-learning decision support system for real-time order sequencing and machine scheduling
Duration: 01.04.2020 bis 31.03.2022
The research project SENECA pursues the development of a self-learning decision support system for real-time order sequencing and machine scheduling. The research question is how machine learning (ML) methods must be applied in order to calculate admissible solutions with sufficient quality for order sequencing and machine allocation problems in real time. Various ML methods are to be investigated with regard to their applicability for order sequencing and machine allocation planning. Due to the highly dynamic nature of modern production systems and the resulting planning uncertainty, it is expected that production sequence planning in particular will benefit from ML-based, real-time capable and adaptive decision support systems. ML algorithms are currently primarily used for regression and classification problems. Their direct use for calculating optimization problems has hardly been researched and industrial applications are not yet known. The technical objective of the project is to develop a software and hardware prototype that supports decision-makers in production planning and control. The technical challenges relate in particular to aspects of production and application-specific design. On the one hand, a high level of user-friendliness is important. This implies, among other things, that humans are always the final decision-making authority. The system should be able to continuously improve itself with human expertise. On the other hand, the assistance system must be designed in such a way that the real-time capability of the solution processes is fully utilized. Proposed order sequences and machine assignments must be able to be transferred from production planning to production control at short notice.
2024
Peer-reviewed journal article
Resource-efficient Edge AI solution for predictive maintenance
Artiushenko, Viktor; Lang, Sebastian; Lerez, Christoph; Reggelin, Tobias; Hackert-Oschätzchen, Matthias
In: Procedia computer science - Amsterdam [u.a.] : Elsevier, Bd. 232 (2024), S. 348-357
2023
Book chapter
AI Engineering als interdisziplinäres Einführungsmodul zwischen Künstlicher Intelligenz und Ingenieurwesen
Lang, Sebastian; Siegert, Ingo; Artiushenko, Viktor; Schleiss, Johannes
In: Informatik 2023 - Berlin : Gesellschaft für Informatik e.V. ; Klein, Maike, S. 381-384 - (GI-Edition. Proceedings; volume P-337) [Tagung: Informatik 2023, Berlin, 26. - 29. September 2023]
Ereignisdiskrete Modellierung autonomer Transportfahrzeuge mittels Open-Source Software - Discrete-event modelling of autonomous transport vehicles using open-source software
Artiushenko, Viktor; Müller, Marcel; Reggelin, Tobias; Lang, Sebastian
In: Simulation in Produktion und Logistik 2023 / ASIM-Fachtagung Simulation in Produktion und Logistik , 2023 - Ilmenau : Universitätsverlag Ilmenau ; Bergmann, Sören *1979-*, S. 271-280 [Tagung: 20. ASIM Fachtagung Simulation in Produktion und Logistik, Ilmenau, 13-15. September 2023]